Artificial Intelligence in Healthcare: Transformations in 2025
- Part 1 — Introduction
- Part 2 — Diagnostic Applications
- Part 3 — Treatment Planning and Management
- Part 4 — Administrative and Operational Uses
- Part 5 — Ethical and Regulatory Considerations
- Part 6 — Education and Training
- Part 7 — Recent Developments (2025–2026)
- Part 8 — Challenges and Limitations
- FAQ — Artificial Intelligence in Healthcare: Your Questions Answered
- Conclusion
Introduction
Have you ever wondered how much of the healthcare you experience is already shaped by artificial intelligence (AI)? If you think of AI as something straight out of sci-fi movies — robots replacing doctors or futuristic machines running hospitals autonomously — you’re not alone. But in reality, AI’s role in healthcare is far more nuanced, fascinating, and impactful than that. By 2025, AI is no longer a distant concept but an integral part of the way medicine is practiced, administered, and evolved. But how did we get here, and why does AI matter so much in healthcare today?
In fact, some of the earliest breakthroughs in AI-driven diagnostics were seen in routine tools like complete blood counts (CBCs), where algorithms began flagging cancer markers earlier than traditional screening methods—a concept explored further in our piece on CBC and Cancer Detection.
What Exactly Is AI, and Why Should We Care About It in Healthcare?
Let’s start with the basics. Artificial Intelligence, simply put, is the development of computer systems that can perform tasks typically requiring human intelligence. Think pattern recognition, learning from data, decision-making, and even natural language understanding. In healthcare, these “intelligent” systems can analyze vast amounts of information, much faster and often more accurately than a human ever could.
Why is this important? Well, healthcare has always been data-heavy. From patient histories to imaging scans, lab results, and real-time monitoring devices, doctors face mountains of data daily. The challenge? Making sense of all this information quickly and correctly to provide the best possible care. This is where AI steps in—not to replace doctors, but to augment their abilities, helping them make better decisions and freeing them from routine tasks.
A Quick Walk Down Memory Lane: How Technology Became Part of Healthcare
It’s worth pausing to appreciate the journey that led to AI’s current role in healthcare. Technology has been a part of medicine for centuries — from the stethoscope invented in the 19th century to the first X-ray machines in the early 20th century. Each innovation transformed how doctors diagnose and treat disease.
Fast forward to the late 20th century and early 2000s, digital health records and basic computer-assisted diagnostic tools became common. But those were just the opening acts. The real revolution began with advances in machine learning and data science. Suddenly, computers could “learn” from data, detect patterns invisible to the human eye, and improve their own performance over time. The result? More sophisticated AI tools designed specifically for medical applications.
Are We Ready for AI? The Growing Role of AI in Modern Healthcare
By 2025, AI is no longer confined to research labs or experimental trials. It’s embedded in everyday healthcare in ways you might not even notice. For example, many radiologists now use AI to help interpret medical images faster and more accurately, improving early detection of cancers or other conditions. Hospitals use AI-driven systems to optimize patient flow and reduce waiting times, making the experience smoother for patients and staff alike.
But the potential goes far beyond these applications. AI is shaping personalized treatment plans, monitoring chronic diseases remotely, assisting with surgical procedures, and even predicting outbreaks of infectious diseases. The promise is enormous — but so are the questions and challenges. How do we ensure AI decisions are fair and unbiased? What happens when AI makes a mistake? How do healthcare providers and patients trust these new tools?
Engaging You: Where Do You See AI in Your Own Healthcare Experience?
Before we dive deeper into AI’s specific applications, take a moment to think about your own experiences with healthcare. Have you ever interacted with a symptom checker app or received personalized health recommendations online? Maybe you’ve had a medical image taken—did you know AI likely played a part in analyzing that image behind the scenes?
This book (or article series) will take you through the fascinating landscape of AI in healthcare today and tomorrow. We’ll explore the breakthroughs, the practical uses, the ethical dilemmas, and the very real challenges of integrating AI into the world of medicine. Whether you’re a healthcare professional, a tech enthusiast, or simply curious about the future of medicine, there’s something here for you.
Diagnostic Applications
Have you ever been curious about how doctors figure out exactly what’s wrong when you walk into a clinic feeling unwell? Diagnosing diseases is part detective work, part science, and increasingly, part AI magic. Diagnosis is often the critical first step in healthcare—get it right, and treatment can begin quickly and effectively; get it wrong, and it can mean delays, mismanagement, or worse. So how is AI changing this cornerstone of medicine? Let’s dig in.
How Does AI See What Humans Can’t? The Rise of AI in Medical Imaging and Pathology
Imagine a radiologist staring at hundreds of chest X-rays or MRIs every day. It’s tedious, time-consuming, and even the most experienced doctors can miss subtle clues. Here’s where AI shines. AI systems, especially those based on deep learning, are trained on thousands or even millions of medical images. Over time, they learn to recognize patterns that might be too subtle or complex for human eyes.
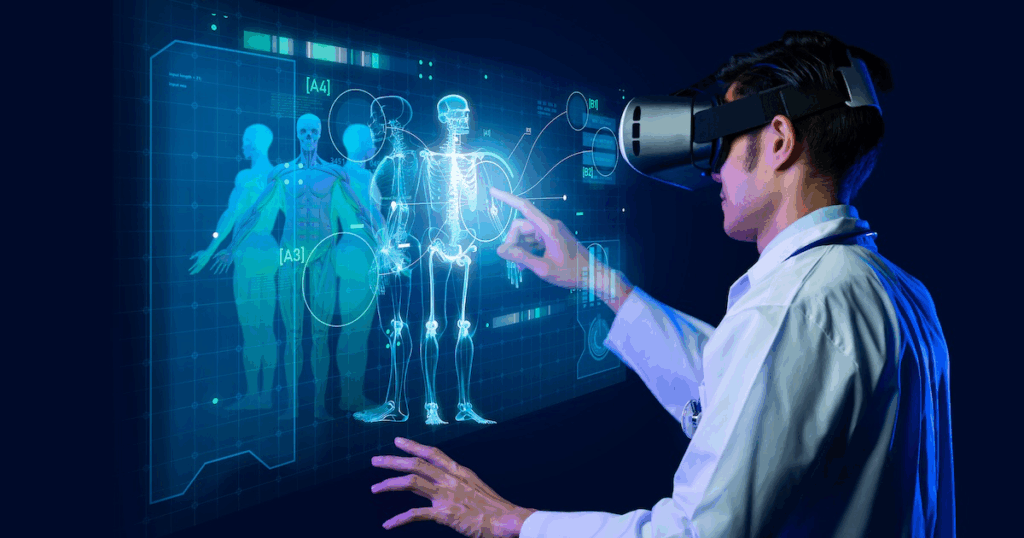
For example, AI algorithms can highlight tiny nodules on a lung scan that might indicate early-stage cancer — something that could easily be overlooked in a busy hospital setting. They can also differentiate between benign and malignant lesions with impressive accuracy. But it’s not just about seeing abnormalities; AI can prioritize urgent cases by flagging the most suspicious images for immediate review by a specialist. This kind of triage helps hospitals manage workload and speeds up diagnosis when time matters most.
Pathology, the study of tissue samples under a microscope, is also benefiting enormously. Traditionally, pathologists examine slides manually—a process requiring years of training and intense concentration. AI-powered digital pathology platforms can scan entire slides and analyze cellular patterns far faster. These tools can even quantify tumor markers or detect genetic mutations, providing additional layers of diagnostic insight.
What’s Changed in Accuracy and Efficiency? More Than Just Faster Results
You might be thinking, “Sure, AI can do things fast, but is it actually better?” The answer, increasingly, is yes. Studies have shown that in certain diagnostic tasks, AI matches or even exceeds the accuracy of expert clinicians. For instance, in breast cancer screening, some AI systems have reduced false positives and false negatives, meaning fewer unnecessary biopsies and fewer missed cancers.
Efficiency gains are also staggering. AI can process and analyze data at speeds no human can match, enabling faster turnaround times. That’s huge in emergency settings or when early intervention can save lives. Plus, AI reduces the burden of repetitive tasks, allowing specialists to focus on complex cases requiring human judgment.
But AI isn’t perfect. It’s important to remember that these systems rely heavily on the quality and diversity of data they’re trained on. If training data is biased or limited, AI might underperform in certain populations or rare conditions. This is why human oversight remains critical—AI should support, not replace, the clinician’s expertise.
Can AI Help Where Doctors Struggle Most?
One exciting area is in diagnosing rare diseases or conditions with ambiguous symptoms. These cases often require years of investigation and multiple specialists. AI can sift through electronic health records, lab results, genetic information, and medical literature to suggest possible diagnoses that might never occur to a doctor working under time pressure. This “clinical decision support” can speed up diagnosis and guide targeted testing.
Moreover, AI is making strides in non-invasive diagnostics. From analyzing voice patterns for signs of neurological diseases to using wearable sensors that continuously monitor vital signs, AI offers novel ways to detect problems before they become critical. For instance, some AI apps analyze a patient’s cough sounds or breathing patterns to screen for respiratory diseases remotely — an especially useful tool in rural or resource-limited settings.
These tools are even beginning to show promise in areas traditionally seen as challenging to diagnose early—such as developmental and neurobehavioral disorders. As discussed in our feature on Autism in 2025, AI is being explored for its ability to detect early signs of autism through behavioral cues, speech analysis, and eye-tracking data.
How Does This Affect You as a Patient or Caregiver?
You might wonder, “Will my doctor trust an AI’s diagnosis?” Or maybe you ask, “Should I rely on symptom checkers or AI apps I find online?” These are important questions. In practice, AI acts like a highly skilled assistant in the diagnostic process. It offers additional perspectives and highlights areas that deserve closer attention. But final decisions, including discussing results with patients, remain a human responsibility.
And yes, AI is increasingly embedded in consumer health apps — but with caution. Not all apps are created equal, and regulatory oversight is still catching up. The best use of AI diagnostic tools combines clinical validation, transparency, and clear communication.
Looking ahead, AI’s diagnostic capabilities will only deepen. Imagine AI integrating data not just from images or lab tests but from genetics, lifestyle trackers, social determinants of health, and even environmental factors. Such holistic views could revolutionize how diseases are detected early, managed, and even prevented.
But as AI grows smarter, questions about accountability, transparency, and fairness grow louder. How do we know the AI is working fairly across all patient groups? How can clinicians explain AI-driven diagnoses to patients in understandable terms? These challenges remind us that AI in diagnosis isn’t just a technical problem — it’s a human one.
Treatment Planning and Management
After nailing down a diagnosis, the next big step is figuring out the best way to treat the condition—and then managing that treatment over time. It’s no secret that treatment plans aren’t one-size-fits-all. Each patient brings unique genetics, lifestyles, and health histories to the table. That’s exactly where AI is proving to be a game-changer: helping tailor treatments to fit individual needs rather than relying on broad averages.
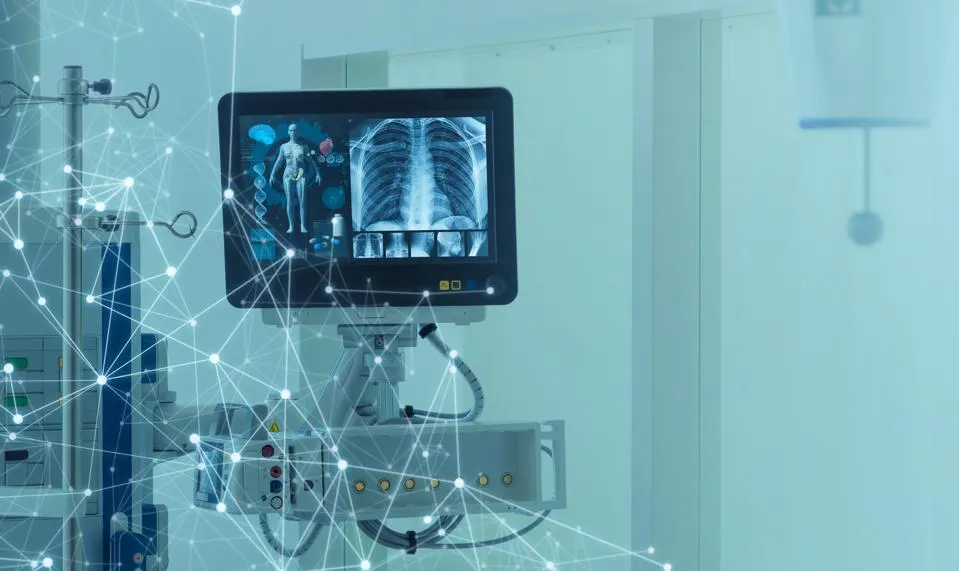
You’ve probably heard the term “personalized medicine” thrown around a lot. It’s more than a buzzword—it’s a fundamental shift in how medicine approaches care. Imagine two patients with the same diagnosis but very different genetic markers or responses to medication. Traditional protocols might treat them identically, but AI digs deeper, analyzing vast datasets to predict which therapy will work best for each person. This isn’t just theory; it’s becoming standard practice in many specialties, improving outcomes and reducing side effects.
But personalized treatment isn’t just about picking the right drug or procedure—it’s also about continuous adjustment. Think about how often you hear about patients needing multiple appointments, tests, and changes in medication. AI helps streamline this by monitoring patients remotely through wearables and smart devices. This constant flow of data allows algorithms to spot early warning signs or shifts in health that might be missed between visits. In real time, treatment plans can be adjusted before a small issue becomes a big problem.
Treatment decisions, especially for complex diseases like cancer, involve juggling countless variables: potential benefits, side effects, patient preferences, and costs. AI supports clinicians by synthesizing all this information—medical literature, trial data, and patient history—offering evidence-based recommendations. The beauty is that these systems don’t replace the doctor’s judgment; instead, they enhance it, providing a clearer picture to guide nuanced discussions with patients.
Of course, there are still challenges. AI’s recommendations are only as good as the data fed into it, and healthcare data can be messy or incomplete. Moreover, the human side of medicine—the emotions, fears, and personal values—can’t be fully captured by algorithms. That’s why AI is best seen as a powerful tool in the hands of compassionate, skilled providers rather than a decision-maker standing alone.
For patients, this means a more dynamic, responsive approach to care. Imagine treatment plans that evolve with you, supported by continuous monitoring and intelligent adjustments that keep you on the best path. The technology doesn’t replace the human touch but aims to enrich it, helping you and your care team make smarter, more informed choices together.
Looking ahead, the future promises even more integration. AI might soon help design new drugs tailored to your genetic profile or run adaptive clinical trials that learn and pivot in real time. It’s a future where medicine isn’t static but a constantly evolving journey—powered by data, guided by expertise, and centered entirely on the individual.
Administrative and Operational Uses
When we think about AI in healthcare, it’s easy to picture high-tech machines diagnosing diseases or suggesting treatment plans. But AI’s impact goes well beyond the exam room or operating theater. Behind the scenes, healthcare systems are incredibly complex machines themselves, juggling patient scheduling, medical records, billing, supply chains, and much more. If you’ve ever wondered why hospital visits sometimes feel chaotic or paperwork overwhelming, you’re tapping into one of healthcare’s biggest challenges.
This is where AI steps in as a silent powerhouse, streamlining these vast administrative and operational tasks that often slow down care delivery. Imagine a hospital’s scheduling system—a seemingly mundane but critical operation. Without optimization, patient appointments, staff shifts, and resource availability can quickly clash, leading to delays, missed appointments, or overworked staff. AI algorithms analyze historical data, patient flow patterns, and staff availability to create smarter schedules that reduce wait times and make sure the right specialists and equipment are ready when needed.
Electronic Health Records (EHRs) are another prime example. Most clinicians spend a significant chunk of their day wrestling with digital charts—typing notes, searching for test results, or ensuring records are complete. AI-powered tools can ease this burden by automating routine documentation tasks or even summarizing patient histories quickly. Instead of drowning in paperwork, doctors get more time to focus on what they do best: patient care.
You might not realize it, but AI also helps hospitals predict patient admissions and bed availability, crucial for managing resources during busy times like flu season or unexpected emergencies. By forecasting these trends, hospitals can proactively allocate staff, stock supplies, and even delay non-urgent procedures if necessary. This kind of foresight helps avoid overcrowding and ensures patients receive timely care.
Billing and coding, notorious for being complex and error-prone, are increasingly handled by AI systems that scan records for accuracy, reducing costly mistakes and speeding up reimbursements. The ripple effect? Less frustration for patients dealing with insurance claims and fewer headaches for healthcare providers.
But with AI managing so many moving parts, you might wonder about privacy and security. After all, administrative data often includes sensitive patient information. Healthcare organizations must balance leveraging AI’s benefits with protecting this data from breaches or misuse. That’s an ongoing challenge requiring robust safeguards, transparent policies, and constant vigilance.
All these behind-the-scenes improvements might seem invisible to patients at first, but they directly impact the quality and efficiency of care you receive. Faster appointments, smoother check-ins, and better communication are all byproducts of AI’s operational power.
Looking forward, AI could drive even more radical changes. Imagine virtual assistants that help patients navigate healthcare systems, personalized reminders based on your health needs, or AI-enabled supply chains that ensure critical medications are always in stock.
The big takeaway? AI isn’t just a tool for doctors and researchers—it’s transforming the very infrastructure of healthcare, making systems smarter, leaner, and more patient-centered than ever before.
Ethical and Regulatory Considerations
As AI becomes more woven into the fabric of healthcare, it raises important questions that go beyond technology—questions about ethics, privacy, fairness, and trust. You might not always see these issues on the surface, but they’re vital to understand if AI is going to be a force for good in medicine.
Think about this: AI systems are only as unbiased as the data they learn from. If the training data reflects existing inequalities—say, underrepresentation of certain racial groups or socioeconomic classes—then the AI might inadvertently reinforce those biases. That could lead to unequal treatment recommendations or misdiagnoses for marginalized communities. It’s a real concern, and one that the healthcare industry is grappling with seriously. How do we ensure AI treats everyone fairly, regardless of background? That’s a question researchers, clinicians, and policymakers are wrestling with every day.
Privacy is another huge consideration. AI thrives on data—massive amounts of it. But healthcare data is deeply personal, often including not just medical history but genetic information, lifestyle details, and more. How do we protect this sensitive information while still allowing AI systems to learn and improve? Regulations like HIPAA in the U.S. and GDPR in Europe provide frameworks, but AI introduces new complexities. For example, if AI tools are developed by private companies, how transparent are they about data usage? Are patients fully informed about how their data is shared and secured? These questions are becoming central to public trust.
And then there’s accountability. When AI helps make a diagnosis or suggests a treatment, who is ultimately responsible if something goes wrong? The doctor? The hospital? The AI developer? This isn’t just a legal puzzle—it’s an ethical one. Patients need to know that behind every recommendation, there’s human oversight and a clear path to address errors or harm.
Because of these challenges, regulators worldwide are stepping up efforts to create guidelines and standards specific to AI in healthcare. These frameworks aim to ensure safety, efficacy, fairness, and transparency. But regulation is a moving target—technology evolves quickly, and laws must keep pace without stifling innovation.
For patients and providers alike, understanding these ethical and regulatory landscapes is crucial. It’s not enough for AI to be powerful—it must be trustworthy and aligned with core values of medicine: respect, beneficence, and justice.
As you interact with healthcare systems increasingly influenced by AI, it’s worth considering these questions: How comfortable are you with AI’s role in your care? What kinds of protections do you expect? How should transparency be balanced with innovation?
Looking forward, the hope is that ongoing dialogue among technologists, ethicists, clinicians, and patients will shape AI systems that are not only cutting-edge but also ethical and fair—building a future where technology enhances humanity rather than complicates it.
This systemic integration of AI isn’t limited to the clinic. Broader strategies for Infectious Disease Preparedness now increasingly rely on AI tools for early detection, resource planning, and outbreak modeling—highlighting the need for ethical AI governance on both individual and population scales.
Education and Training
We often focus on the technology itself, but what about the people behind the scenes? After all, even the smartest AI tools won’t make a difference unless healthcare professionals know how to use them effectively. So how is education adapting to prepare doctors, nurses, and other medical staff for a world where AI is an everyday partner?
The truth is, the integration of AI into healthcare demands a shift in training that goes far beyond simply teaching clinicians how to click buttons or interpret AI outputs. It’s about fostering a mindset that embraces new tools while critically evaluating their strengths and limitations. This requires a blend of technical skills, ethical understanding, and communication strategies.
Medical schools and training programs have begun incorporating AI-related content into their curricula, but it’s still early days. Imagine a doctor learning not only about anatomy and pharmacology but also about machine learning basics, data literacy, and the potential biases of algorithms. This knowledge empowers clinicians to engage actively with AI tools rather than passively accepting their outputs.
But it’s not just physicians. Nurses, technicians, administrators, and even policymakers need training tailored to their roles. Nurses, for example, may use AI-driven monitoring systems to detect early signs of patient deterioration, requiring them to understand how alerts are generated and when to escalate care. Meanwhile, healthcare leaders must grasp AI’s operational and ethical implications to make informed decisions on adoption and governance.
What about continuing education? AI evolves rapidly, and initial training can’t be the end of the story. Ongoing professional development, workshops, and interdisciplinary collaboration will be key to keeping skills current and fostering a culture of learning.
Beyond formal education, there’s also the question of public literacy. As patients increasingly encounter AI-driven tools—from symptom checkers to personalized health apps—how do we ensure they understand these technologies? Patient education can help demystify AI, build trust, and encourage more informed engagement with digital health.
Ultimately, preparing the healthcare workforce for AI isn’t just about technology—it’s about people. It’s about blending human judgment with machine intelligence in a way that enhances care and respects the values at the heart of medicine.
Recent Developments (2025–2026)
By 2025 and into 2026, AI in healthcare is no longer just a promising concept—it’s delivering tangible breakthroughs across multiple specialties. You might be surprised how quickly some of these advances are moving from research labs into everyday clinical practice. The pace of innovation is staggering, and the results are already improving patient outcomes in meaningful ways.
Take oncology, for example. AI-powered tools are now capable of integrating genetic data, imaging results, and patient history to craft personalized cancer treatment plans with greater precision than ever before. Some hospitals have reported faster treatment initiation and better survival rates, all thanks to algorithms that can predict how tumors will respond to specific therapies. This isn’t just improving outcomes—it’s giving patients hope where there was uncertainty before.
In cardiology, AI-driven wearable devices have become more sophisticated at detecting arrhythmias and predicting heart failure episodes days before symptoms become obvious. For patients managing chronic heart conditions, this means earlier intervention and fewer emergency hospitalizations. These advances also allow cardiologists to monitor large patient populations remotely without overwhelming clinic schedules.
Neurology has seen exciting developments too, with AI systems analyzing speech patterns and movement to detect early signs of diseases like Parkinson’s and Alzheimer’s. Early diagnosis is crucial for managing these conditions, and AI’s ability to spot subtle changes is opening new doors for intervention and research.
Even beyond individual specialties, AI is making a difference in public health. Predictive models are better anticipating disease outbreaks, helping governments and healthcare organizations mobilize resources proactively. This was particularly evident during recent flu seasons and emerging infectious diseases, where AI-enhanced surveillance helped contain spread and optimize vaccination campaigns.
These recent developments aren’t just technical marvels—they’re changing real lives. Case studies abound showing how AI-assisted diagnostics led to earlier cancer detection, how remote monitoring reduced heart failure admissions, and how AI-driven workflows increased clinic efficiency, allowing providers to see more patients with better outcomes.
But perhaps what’s most exciting is how these innovations feed back into each other. Advances in one field often inspire breakthroughs elsewhere, creating a virtuous cycle of improvement. As AI systems become smarter and more integrated, their collective impact grows exponentially.
Still, with all this progress, it’s important to remain grounded. While AI tools are improving rapidly, they’re part of a broader ecosystem that includes human expertise, patient engagement, and systemic healthcare factors. The best results come from thoughtful integration, where technology complements rather than replaces human care.
As you think about these breakthroughs, consider how they might affect your own healthcare experience or that of your loved ones. What if your doctor could catch a serious condition weeks earlier thanks to AI? How might remote monitoring change chronic disease management for you or someone you know? These questions highlight the exciting promise—and the ongoing journey—of AI in healthcare.
Challenges and Limitations
As exciting as all these AI breakthroughs are, it’s crucial to take a step back and look at the full picture—including the bumps in the road. No technology is perfect, especially when it’s navigating something as complex and deeply human as healthcare. So, what are the real challenges and limitations AI faces today?
First off, technical hurdles remain significant. AI systems require vast amounts of high-quality data to perform well, but healthcare data is often fragmented, incomplete, or siloed across different institutions. This fragmentation makes it tough to train AI models that are truly representative and reliable. If an AI system has only seen data from one hospital or one demographic group, it might struggle or even fail when applied elsewhere.
Another tricky area is interpretability. Many AI algorithms, especially deep learning models, operate like “black boxes” — they produce results, but it’s often unclear exactly how or why they reached those conclusions. For clinicians, this lack of transparency can be frustrating and a barrier to trust. After all, it’s hard to base life-or-death decisions on a recommendation you can’t fully understand or explain to your patient.
Human oversight is a critical piece of the puzzle. AI should never be seen as a replacement for doctors but as a tool to assist them. Balancing this partnership is easier said than done. Over-reliance on AI might lead to deskilling or complacency, while underutilization wastes valuable potential. Finding that sweet spot requires ongoing training, cultural shifts in healthcare settings, and thoughtful integration strategies.
Ethical challenges also persist. Bias in AI remains a real and pressing problem. Without careful design and monitoring, AI can perpetuate inequalities or make decisions that unfairly disadvantage certain groups. Addressing these issues demands not just technical fixes but broader systemic efforts to diversify data, improve transparency, and involve diverse stakeholders in AI development.
Then there’s the regulatory and legal landscape, which is still catching up. Clear standards for safety, effectiveness, and accountability are essential, but regulations must balance innovation with patient protection. The pace of AI development often outstrips the speed of policymaking, leaving gaps that can cause uncertainty for providers and patients alike.
Lastly, let’s not forget the human element—patients’ trust and acceptance. AI’s complexity and perceived impersonality can be intimidating. Patients want to feel heard and understood, not just processed through algorithms. Ensuring that AI enhances rather than detracts from the human connection in medicine is a subtle but vital challenge.
Despite these hurdles, progress continues steadily. Each challenge is a call to refine AI technologies, improve education, and develop smarter policies. The future of AI in healthcare will depend as much on solving these limitations as on advancing the technology itself.
As you consider AI’s role in your healthcare journey, it’s important to appreciate both its promise and its current boundaries. Awareness of these challenges can help foster realistic expectations and encourage constructive conversations about how to best harness AI’s power while safeguarding quality and equity.
Artificial Intelligence in Healthcare: Your Questions Answered
1. What exactly is AI, and why is it important in healthcare?
AI refers to computer systems designed to perform tasks that typically require human intelligence, like recognizing patterns and making decisions. In healthcare, AI helps process massive amounts of data quickly and accurately, supporting doctors in diagnosis, treatment planning, and operations, ultimately improving patient care.
2. How is AI improving diagnostic accuracy?
AI can analyze medical images and pathology samples with great precision, often spotting subtle signs that human eyes might miss. This leads to earlier and more accurate diagnoses, such as detecting cancers or rare diseases faster than before.
3. Can AI personalize treatment plans?
Yes. By analyzing a patient’s genetic data, lifestyle, and treatment response, AI helps create tailored therapies that optimize effectiveness and minimize side effects, moving away from a one-size-fits-all approach.
4. How does AI assist in treatment monitoring and adjustment?
Wearables and remote monitoring tools combined with AI track patients’ health in real time, allowing doctors to adjust treatments proactively before complications arise.
5. What role does AI play in hospital administration?
AI streamlines scheduling, manages electronic health records, predicts patient admissions, and automates billing—making healthcare systems more efficient and reducing wait times for patients.
6. What are the ethical concerns surrounding AI in healthcare?
Key issues include data privacy, potential bias in AI algorithms, transparency, and accountability for AI-driven decisions. Ensuring fairness and protecting sensitive data are major priorities.
7. Are healthcare professionals being trained to work with AI?
Training programs are evolving to include AI literacy, helping clinicians understand and critically evaluate AI tools. Continuous education is essential to keep pace with rapid technological advances.
8. What are some recent breakthroughs in AI healthcare applications?
Recent developments include AI-assisted cancer treatment planning, wearable devices predicting heart failure episodes early, and AI systems detecting neurological disorders through speech and movement analysis.
9. What are the biggest challenges facing AI in healthcare today?
Challenges include data quality and fragmentation, AI transparency, regulatory gaps, risk of bias, and balancing AI use with human oversight to maintain trust and effectiveness.
10. How will AI impact patients directly in the near future?
Patients can expect more personalized care, faster diagnoses, proactive treatment adjustments, and smoother interactions with healthcare systems through AI-powered tools—all while maintaining the human connection with their providers.
Conclusion
Artificial intelligence in healthcare is no longer a futuristic vision—it’s very much a present reality transforming how medicine is practiced and experienced. From boosting diagnostic accuracy to personalizing treatments, streamlining hospital operations, and helping healthcare professionals make better decisions, AI’s impact in 2025 is broad and profound.
Yet, as with any powerful tool, the benefits come alongside important challenges. Ethical considerations, data privacy, fairness, and the need for human oversight remind us that AI is not a panacea but a partner in care. The most successful healthcare systems will be those that blend technological innovation with empathy, transparency, and rigorous governance.
For patients, clinicians, and policymakers alike, the journey with AI in healthcare invites curiosity, caution, and collaboration. As we move forward, the hope is clear: AI will help us deliver smarter, more equitable, and more compassionate care—shaping a future where technology amplifies humanity’s best qualities rather than replacing them.
So whether you’re a patient wondering how AI might affect your next doctor’s visit, a healthcare professional preparing for new tools in your practice, or simply curious about this exciting frontier, the story of AI in healthcare is one of ongoing discovery. And as you’ve seen, it’s a story that’s just getting started.